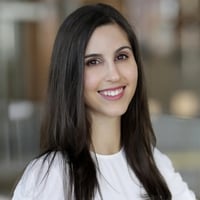
Hiring and firing – what if AI made the call?

Various government inquiries are closely examining the impacts of AI-systems performing human resources functions, and it is only a matter of time before these technologies are regulated.
Artificial intelligence (AI) has entered the HR space. With growing economic pressures, AI can present as an attractive option for employers to reduce costs and increase efficiency. AI technologies now offer support for a range of human resources functions such as recruitment, performance reviews, rostering, allocation of tasks, and termination of employment. In fact, several vendors selling people management technologies have reported significant increases in sales since the COVID-19 pandemic.
AI-systems range from reactive tools that automate routine administrative tasks like payroll processing and leave management, to more sophisticated automated decision-making systems that use machine learning algorithms to assess information. This includes matching candidates to job descriptions, screening candidates for recruitment, and profiling employees for performance reviews, rostering and wage increases. Some software also offer the ability to monitor employees' computers and send automatic violation notices when employees are late, idle, absent or unproductive.
A recent evolution is the integration of generative AI-systems, which can produce written content ranging from job descriptions to performance evaluation narratives. When used to power chatbots, these AI applications can also engage in natural language interactions with candidates and employees. AI-powered large language models can undertake preliminary candidate screening, where candidates' applications are assessed against role-specific criteria to generate shortlists. These tools are also used for performance appraisals and promotion plans, where AI-systems collect and analyse vast quantities of data from various internal sources based on fixed criteria. For instance, IBM has developed AI-powered systems that draft preliminary performance summaries by synthesising documented achievements, client feedback, and KPI attainment data.
Inquiries into the use of AI
Governments around the world are now beginning to grapple with how to regulate AI. In August last year, the European Union's Artificial Intelligence Act 2024 (EU Act) came into force, which has categorised the use of AI-systems in employment and workers' management as "high risk". The EU Act has set out strict compliance measures in this area, including establishment of a risk management system to identify, assess, and mitigate risks throughout the lifecycle of the AI-system.
The Australian Government's House Standing Committee on Employment, Education and Training has also recommended that AI-systems used for employment related purposes are classified as high risk. The House Committee's The Future of Work – Inquiry into the Digital Transformation of Workplaces report was tabled earlier this week. It is preceded by:
- the Department of Industry, Science and Resources' Proposals Paper for introducing mandatory guardrails for AI in high-risk settings (September 2024); and
- the final report of the Senate's Select Committee on Adopting Artificial Intelligence, which inquired into the opportunities and impacts arising out of the uptake of AI technologies across various industries and sectors in Australia (November 2024).
Issues arising from use of AI-assisted systems in human resources
Like any emerging technology, while AI has the potential to significantly increase efficiency and reduce costs for employers, there are inherent legal and practical risks involved with its usage. We are yet to fully understand how AI-systems will interact with our existing employment, work health safety, and anti-discrimination legislation framework. However, as AI-systems are often used across vast amounts of data, a single error or embedded bias in an AI model can potentially have wide ranging ramifications – not only in terms of the unfairness caused to individuals, but also in terms of exposure to regulatory measures, penalties, legal costs, and damages for businesses that implement the system.
Inherent bias
Submissions to the Senate Committee recognised that inherent biases can occur in connection with AI-assisted decision making due to embedded biases within datasets used to train AI models. This includes the under- and over- representation of certain social groups within these datasets and an inability to effectively screen or analyse data in relation to workers requiring special consideration, for instance those from non-English speaking backgrounds or with disability. It was acknowledged that this could entrench unfairness or discrimination in decision-making at a large scale.
Workplace surveillance
Contemporary AI-systems can also support audiovisual and computer surveillance data-gathering. Employers can gather information on facial expressions in video calls, tones of voice in customer interactions, data from wearable devices, and physical movements within the work environment, and use AI-systems to generate detailed operational profiles of employees and customers. "New workforce optimisation technologies" can monitor workers at their desks, pre-determining "non-compliant" behaviour based on keystroke tracking, taking screenshots of an employee’s computer, or video recording them at their desk. The House Committee has recognised deficiencies in employment and surveillance legislation where, although worker consent for surveillance may have been obtained, there do not appear to be any limits as to how such surveillance data may be used.
Performance assessment and rostering
Submissions to the House Committee have expressed concerns over the use of AI-systems in assessing employee performance. Certain AI-systems may lead to managers drawing incorrect inferences of employees' performance. For example (as provided in the House Committee's report), if a customer makes a comment to a worker such as, "unfortunately, the weather isn’t great" during a recorded conversation, the technology is trained to pick up the word "unfortunately" and rate the customer interaction as negative.
It is also suggested that excessive monitoring and surveillance may lead to significant physical and psychosocial harms, due to workers feeling pressured to achieve unreasonable KPIs and an increase in opportunities for bullying and inappropriate conduct.
QUT Centre for Decent Work & Industry submitted to the House Committee that early indications from its research suggest that, where AI-systems are used for rostering and assessing employee performance, workers may be impacted if due consideration is not given to "skill variety, task significance and task identity". For example, in retail, workers may find it difficult to complete a task within the allotted timeframe if they are consistently or unexpectedly interrupted by customers. This may lead to incorrect inferences about their performance or reduction in quality of the work in order to meet the quantity of work allotted to them.
AI-led rostering systems may also reduce the scope for consultation with workers, which could impact workers who require special considerations, such as those with disability or carer responsibilities. According to an example provided to the House Committee, an employer who used AI-systems to make roster changes was unable to identify the information that was used by the system to make such changes, which was considered a defect in the consultation process.
Consultation
Submissions to the Senate Committee identified that consultation with workers to help prevent or mitigate bias-related risks in the use of AI is currently insufficient. The House Committee also received submissions with differing views on whether the introduction of AI-systems constitutes a major change that would trigger consultation obligations under the Fair Work Act 2009 (Cth). While the House Committee has not formed a conclusive view on the question, it has recommended that consultation obligations should be strengthened "before, during, and after the introduction of new technology".
Accountability
"Black box" AI-systems are systems whose internal workings are concealed from its users. Users can see the outputs made by the system but are not provided with information on how the system produced the output. For example, an AI-system may evaluate a job candidate’s resume, but may not be able to explain the factors it considered or how it weighed those factors when making such evaluation. Developers may often intentionally conceal internal workings on a system. However, often, this may occur unintentionally due to the complex deep learning processes involved in creating such systems.
The House Committee acknowledged that workers have the right to understand decisions that impact their employment and how they were made. However, due to the "Black Box" often present in AI-systems, it may be difficult to ascertain liability for unlawful conduct facilitated by an AI-system. It also remains unclear whether decisions made by AI-systems would fall within the meaning of a "person" engaging in unlawful conduct under anti-discrimination legislation as well as for the purposes of invoking accessorial liability provisions under various laws, such as the Fair Work Act, anti-discrimination legislation and work health safety legislation.
Recommendations from the House Committee
Completing their inquiries by way of public hearings and submissions, both Committees have made recommendations to the Australian Government, which are largely similar in nature. In addition to the recommendations mentioned above, following are some other key recommendations made by the House Committee:
Adoption of the Proposals Paper's mandatory guardrails, including implementing a new legislation: Introduce AI-specific legislation with a dedicated independent regulator to regulate high-risk uses of AI-systems. The proposed form of legislation is similar to the EU Act and Canada's proposed Artificial Intelligence and Data Act.
Fair Work Act reforms: Review of the Fair Work Act as follows:
- ensure that decision making using AI-systems is covered under the Act and that employers remain liable for such decisions;
- enhance worker entitlements like flexible work arrangements in the National Employment Standards to respond to the adverse effects of significant job redesign caused by emerging technologies;
- ban the use of AI-systems for final decision-making without any human oversight, especially human resourcing decisions;
- introduction of the right to an explanation in relation to decisions made by AI-systems, which is similar to the EU Act; and
- extension of positive equality duties to all protected attributes grounds under the Fair Work Act, as modelled on the positive duty in the Sex Discrimination Act 1984 (Cth).
Privacy reforms: Review of the Privacy Act 1988 (Cth) and the Fair Work Act to inter alia:
- ban high-risk uses of worker data;
- require meaningful consultation and transparency; and
- empower the Fair Work Commission to receive complaints regarding breaches of workers' privacy obligations.
WHS Code of Practice: Address specific work health safety risks associated with the use of AI-systems, especially to mitigate psychosocial risks.
Transparency and accountability: Ensure the developers of AI products are transparent about their use of training datasets, including through use of diverse training sets and conducting regular mandatory independent audits.
What to expect next
Overall, the recommendations under the Proposals Paper, the House Committee's report and the Senate Committee's report support the introduction of regulation to govern AI-systems across various industries and areas of law. The Parliamentary Joint Committee of Public Accounts and Audit is also currently inquiring into the use and governance of AI-systems by public sector entities. The Joint Committee has concluded receiving submissions and holding public hearings, but its report is yet to be published.
Considering the approach taken by the House Committee, the Senate Committee and the Department of Industry, Science and Resources, we expect the Joint Committee's recommendations to be in line with the recommendations outlined above.
What steps should employers take?
Employers should take proactive steps, not only to prepare themselves for upcoming AI regulation, but also to reduce exposure to employment-related claims associated with the implementation of AI-systems. As part of this, employers should:
- consult with workers about the use of AI technology to ensure that their inputs are taken into account to mitigate any risk of inherent bias. Consultation should occur throughout all stages of the technology's deployment, including research, design, data input, training, and piloting of the system.
- regularly assess and test datasets to detect any bias. Failure to consider biological, cultural, religious, and other differences in datasets and how they are analysed can result in legal risk.
- regularly monitor and update AI-systems to correct any biases that may emerge over time.
- ensure there is human intervention at all appropriate stages of the AI-driven process.
- balance the use of surveillance methods for business needs with workers privacy rights.
- seek appropriate technical and legal advice before implementing AI-systems, especially before taking adverse decisions like disciplinary action or termination of employment.
Case studies
There are a number of real-life examples which illustrate the types of issues employers should look out for when implementing AI-assisted systems in human resource management.
- A government agency introduced an AI-assisted selection process for a bulk-round of recruitment. The AI model eliminated the need for a selection panel to review applications and interview candidates. Rather, the AI model assessed candidates based on various online assessments including psychometric tests, questionnaires, and self-recorded video responses, and then placed them in specific merit pools. Approximately 18,000 applications were received, which resulted into two merit pools. The merit pools were used by the agency to fill vacant positions (including the promotions of existing employees) and 747 recruitment decisions (including promotion decisions) were made. The Australian Merit Protection Commissioner received 279 applications challenging the decisions. Of these, 115 individual promotion decisions were reviewed and 11 decisions were overturned. The only basis for overturning such decisions was a finding that the applicant has demonstrated they have more merit than the person who was promoted. According to the Merit Protection Commissioner's report, the high number of overturned decisions indicated that the selection process was not always meeting its key objective, which is to identify and select the most meritorious candidates for the roles advertised. The report also noted that workers with a proven track record were rated unsuitable and ruled out for promotion or permanency because their video recording or written application failed to use language that the AI model's algorithm was trained to identify as being suitable.
- A technology company developed an AI model that was trained to screen candidates and give them a rating between one and five stars. It was discovered that disproportions in the dataset used to train the model led to a self-taught bias, and the rating system then favoured male over female applicants and discriminated against people with carer responsibilities.
- A gig economy platform used automated processes to manage workers' account deactivation processes. Subsequently, the Digital Labour Platform Deactivation Code now requires that a "human representative" of a gig economy platform must consider a worker’s response in relation to a complaint and discuss them with the worker, prior to making any decision.
Get in touch
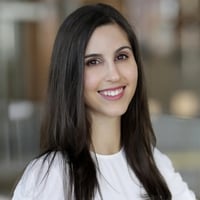